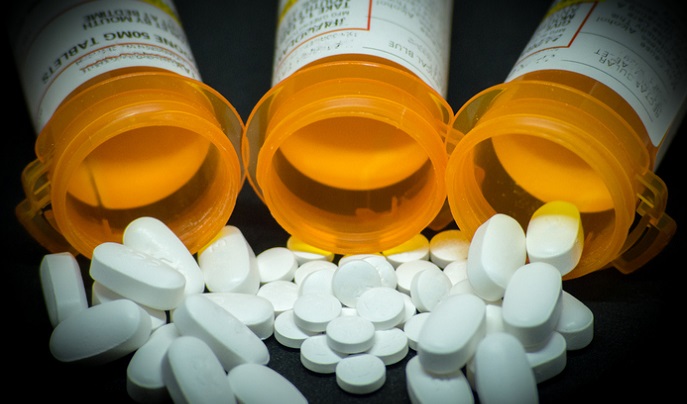
- Machine learning tools can predict the likelihood that a patient with a urinary tract infection (UTI) won’t respond to antibiotics, which could reduce antibiotic resistance in outpatient clinical care.
Antibiotic resistance is a global health and development threat, cautions the World Health Organization (WHO). Antibiotic resistance occurs when bacteria, viruses, fungi, and parasites change over time and no longer respond to medicines, making infections harder to treat and increasing the risk of disease spread, illness, and death.
In a study published in Science Translational Medicine, researchers noted that the issue of antibiotic resistance is particularly significant with UTIs. These infections impact half of all women and add almost $4 billion per year in unnecessary healthcare costs.
To treat UTIs, doctors often prescribe antibiotics called fluoroquinolones, which are inexpensive and generally effective. However, these treatments have also been found to put women at risk of contracting other infections like C. difficile and staph infections. The antibiotics are also associated with a higher risk of tendon injuries and aortic tears, which can be life-threatening.
Medical associations have issued guidelines recommending fluoroquinolones as second-line treatments that should only be used on a patient when other antibiotics are ineffective or have adverse reactions. However, physicians with limited time and resources continue to prescribe these treatments at high rates.
READ MORE: Pharmacist-Led Antibiotic Stewardship Approach Cuts Overprescribing
Researchers from MIT saw this issue as an opportunity to develop a machine learning tool that can help providers make better treatment decisions for their patients.
When a patient comes into the emergency room or their primary care physician’s office with a suspected UTI, even when the infection is confirmed the specific bacteria is still unknown, making it difficult to find a particular treatment path.
The machine learning algorithm makes a recommendation based on EHR data from more than 10,000 patients from Brigham and Women’s Hospital and Massachusetts General Hospital (MGH). The model can predict the probability that a patient’s UTI can be treated by first- or second-line antibiotics, and can then make a recommendation for a specific treatment that selects a first-line agent as frequently as possible, without leading to an excess of treatment failures.
The results of the study showed that the algorithm can allow clinicians to reduce use of second-line antibiotics by 67 percent. For patients where providers chose a second-line drug but the algorithm recommended a first-line drug, the first-line drug ended up working more than 90 percent of the time.
When clinicians chose an inappropriate first-line drug, the algorithm chose an appropriate first-line drug almost half the time.
READ MORE: Urgent Care Centers Show Lackluster Antibiotic Stewardship
The system features a thresholding algorithm that clinicians will hopefully be able to apply to a wide range of drugs that all face a similar challenge of balancing effective treatments with the desire to minimize use of second-line antibiotics. Researchers also designed the model to be integrated directly into the EHR, which will eliminate unnecessary steps and additional workflows.
“With this algorithm we can actually ask the doctor what specific probability of treatment failure they’re willing to risk in order to reduce the use of second-line drugs by a certain amount,” said Sanjat Kanjilal, a Harvard Medical School lecturer, infectious diseases physician and associate medical director of microbiology at the BWH.
The project is part of a larger wave of machine learning models that researchers have developed to predict antibiotic resistance in infectious syndromes such as bloodstream infections and using pathogenic genomic data.
Although many of these approaches provide clinical information, most of these tools haven’t been widely adopted because of their lack of interpretability, difficulty integrating into clinical workflows, and a lack of evidence to prove that they actually work in hospital settings.
The research team hasn’t tested the algorithm on more complicated forms of UTIs that involve pre-existing conditions, and they noted that proof of utility can only be demonstrated through a randomized controlled trial. However, they stated that a wide range of UTI cases are compatible with the system.
READ MORE: Congress Urged to Incentivize Combating Antibiotic Resistance
Going forward, the team will focus their efforts on conducting a randomized controlled trial comparing usual practice to algorithm supported decisions. Researchers will also aim to increase the diversity of their sample size to improve the algorithm’s recommendations across race, ethnicity, socioeconomic status, and more complex health backgrounds.
“What’s exciting about this research is that it presents a blueprint for the right way to do retrospective evaluation,” said Sontag. “We do this by showing that one can do an apples-to-apples comparison within the existing clinical practice. When we say we can reduce second-line antibiotic use and inappropriate treatment by certain percentages, we have confidence in those numbers relative to clinicians.”
"machine" - Google News
November 05, 2020 at 08:30PM
https://ift.tt/2TTrQAo
Machine Learning Tool May Combat Outpatient Antibiotic Resistance - HealthITAnalytics.com
"machine" - Google News
https://ift.tt/2VUJ7uS
https://ift.tt/2SvsFPt
Bagikan Berita Ini
0 Response to "Machine Learning Tool May Combat Outpatient Antibiotic Resistance - HealthITAnalytics.com"
Post a Comment