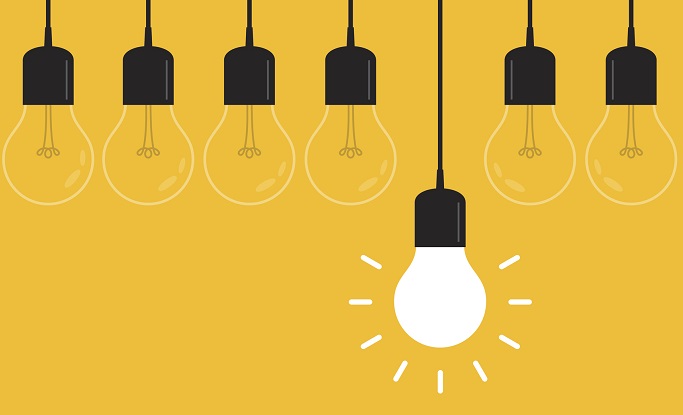
- A new biochip uses machine learning to differentiate between cancers and healthy tissues at the single-cell level, according to a study published in Advanced Biosystems.
Because of the heterogeneous nature of cancer, patients can experience resistance to therapies and different individuals can have varied reactions to the same treatment, researchers noted.
“Cancer cell and tumor heterogeneity can lead to increased therapeutic resistance and inconsistent outcomes for different patients,” said lead author Kushal Joshi, a former UCI graduate student in biomedical engineering.
To better detect and evaluate cancer types, single-cell analysis is critical, but traditional methods can be difficult to perform.
“Single-cell analysis is essential to identify and classify cancer types and study cellular heterogeneity. It’s necessary to understand tumor initiation, progression and metastasis in order to design better cancer treatment drugs,” said senior author Rahim Esfandyarpour, UCI assistant professor of electrical engineering & computer science as well as biomedical engineering.
“Most of the techniques and technologies traditionally used to study cancer are sophisticated, bulky, expensive, and require highly trained operators and long preparation times.”
To overcome these challenges, researchers from University of California, Irvine combined machine learning techniques with accessible inkjet printing and microfluidics technology to develop low-cost, miniaturized biochips that are easy to prototype and can classify multiple cell types.
In the biochip, samples travel through microfluidic channels with carefully placed electrodes that monitor differences in the electrical properties of diseased versus healthy cells in a single pass. The team set out to develop a way to prototype key parts of the biochip in about 20 minutes with an inkjet printer, allowing for easy manufacturing in diverse settings. Most of the materials involved are reusable or disposable if inexpensive.
The biochip uses machine learning to manage the large amount of data the tiny system produces. The algorithms accelerate the processing and analysis of large datasets, aiding in rapid and effective decision-making.
By including machine learning in the development of the biochip, researchers have improved the accuracy of analysis and reduced dependency on skilled analysts, which could also make the technology appealing to researchers in the developing world.
“The World Health Organization says that nearly 60 percent of deaths from breast cancer happen because of a lack of early detection programs in countries with meager resources,” said Esfandyarpour.
“Our work has potential applications in single-cell studies, in tumor heterogeneity studies and, perhaps, in point-of-care cancer diagnostics – especially in developing nations where cost, constrained infrastructure and limited access to medical technologies are of the utmost importance.”
Data analytics tools have played an increasingly significant role in improving cancer treatment and diagnosis. A recent study published in the American Journal of Physiology-Cell Physiology showed that a predictive analytics method was able to detect with 90 percent accuracy which stage 0 breast cancers are likely to spread and recur after surgery.
“The computer is looking for patterns in the images that humans can’t readily discern, from the level of individual pixels up to an entire image of a million pixels,” said Howard Petty, PhD, a professor of ophthalmology and visual sciences, and of microbiology and immunology at Michigan Medicine, the University of Michigan’s academic medical center.
The program is now able to correctly identify aggressive and non-aggressive disease 96 percent of the time.
“That’s pretty impressive when you consider that a human looking at these images would get the answer right about 70 percent of the time,” Petty said. “And we’ve continued to work on reducing the level of false negatives.”
"machine" - Google News
October 08, 2020 at 08:30PM
https://ift.tt/3luuuIL
Machine Learning Helps Classify Breast Cancer Cell Types - HealthITAnalytics.com
"machine" - Google News
https://ift.tt/2VUJ7uS
https://ift.tt/2SvsFPt
Bagikan Berita Ini
0 Response to "Machine Learning Helps Classify Breast Cancer Cell Types - HealthITAnalytics.com"
Post a Comment