WEST LAFAYETTE, Ind. — Computer vision researchers use machine learning to train computers in visually recognizing objects – but very few apply machine learning to mechanical parts such as gearboxes, bearings, brakes, clutches, motors, nuts, bolts and washers.
A team of Purdue University mechanical engineers has created the first comprehensive open-source annotated database of more than 58,000 3D mechanical parts, designed to help researchers apply machine learning to those parts in actual machines.
“We are in the deep learning era, using computers to search for things visually,” said Karthik Ramani, Purdue’s Donald W. Feddersen Distinguished Professor of Mechanical Engineering. “But no one is focusing on the parts that go into machines: pipes, bearings, motors, washers, nuts and bolts, etc. Those are the things that are important to us as engineers and manufacturers. We want to be able to point a camera at a real-world part, and have the computer tell us everything about that part or design.”
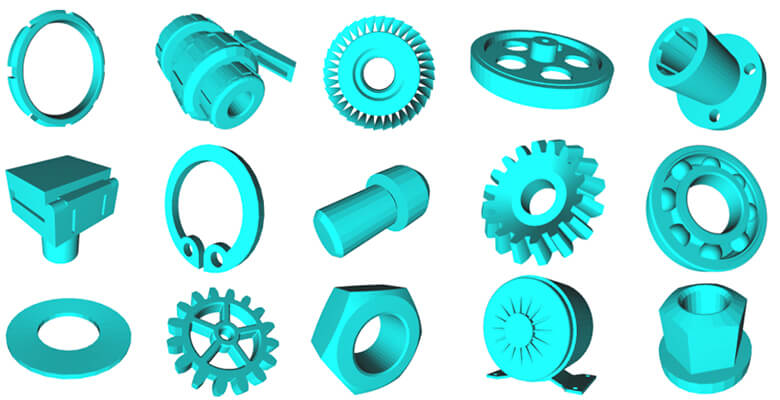
Ramani’s team experimented with visual search for parts in the early 2000s, but computing power and machine learning techniques were not yet sufficiently advanced. In the years since, researchers have learned that building a solid dataset is all about both quality and quantity.
“Deep learning is data hungry,” Ramani said. “It needs a lot of examples for the computer to learn what humans mean and how things relate to each other. That means we needed a lot of 3D models of parts which also required an underlying engineering classification.”
The team began by partnering with a French company called TraceParts, which gave Purdue researchers access to their database of 3D engineering parts. The team collaborated with the University of Texas, Austin assistant professor Qixing Huang to scour other databases for similar 3D models. They ended up compiling a database of 58,696 mechanical components. A video about the database is available on YouTube.
But a database is no good without good data. Ramani’s team organized the parts by establishing a hierarchical taxonomy of 68 classes, based on the International Classification for Standards, a system of technical standards created and maintained by the International Organization for Standardization.
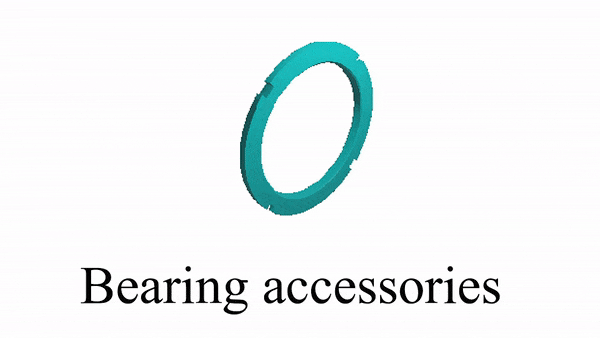
“Now when a computer sees a picture of a seal component, it will know that it fits in the category of dynamic seals and then, more specifically, under composite seals,” Ramani said.
The researchers now have published their open-source database, inviting computer vision and machine learning researchers to access it and create their own experiments. They showcased their work at the 16th European Conference on Computer Vision in August.
With a benchmark dataset now established, what is the future of machine learning for making machines?
“We see many real-world situations for this technology,” Ramani said. “Imagine you’re working maintenance in a factory, and you’re replacing a part of a machine. You can point a camera at the part, and the computer will recognize it, and instantly give you all the specifications of that part – what it’s called, what it connects to, and where they are physically stored in the factory. This could even happen through augmented reality glasses; you could have your company’s entire visual catalog instantly at your fingertips and learn how to fix things or order parts.
“There are a lot of challenges in machine learning. But you can’t deal with the challenge unless you know how to test how well you’re doing. We are proud to have created the first large-scale benchmark dataset of annotated 3D mechanical components in the world, and we hope to advance visual search in the deep learning era.”
This research is being conducted at the Convergence Design Laboratory at Purdue University. The work is partially supported by the National Science Foundation under grants FW-HTF 1839971, OIA 1937036, and CRI 1729486.
About Purdue University
Purdue University is a top public research institution developing practical solutions to today’s toughest challenges. Ranked the No. 5 Most Innovative University in the United States by U.S. News & World Report, Purdue delivers world-changing research and out-of-this-world discovery. Committed to hands-on and online, real-world learning, Purdue offers a transformative education to all. Committed to affordability and accessibility, Purdue has frozen tuition and most fees at 2012-13 levels, enabling more students than ever to graduate debt-free. See how Purdue never stops in the persistent pursuit of the next giant leap at https://purdue.edu/.
Media contact: Kayla Wiles, 765-494-2432, wiles5@purdue.edu
Writer: Jared Pike
Source: Karthik Ramani, ramani@purdue.edu
Journalists visiting campus: Journalists should follow Protect Purdue protocols and the following guidelines:
- Campus is open, but the number of people in spaces may be limited. We will be as accommodating as possible, but you may be asked to step out or report from another location.
- To enable access, particularly to campus buildings, we recommend you contact the Purdue News Service media contact listed on the release to let them know the nature of the visit and where you will be visiting. A News Service representative can facilitate safe access and may escort you on campus.
- Correctly wear face masks inside any campus building, and correctly wear face masks outdoors when social distancing of at least six feet is not possible.
ABSTRACT
A Large-scale Annotated Mechanical Components Benchmark for Classification and Retrieval Tasks with Deep Neural Networks
Sangpil Kim, Hyung-gun Chi, Xiao Hu, Qixing Huang, and Karthik Ramani
We introduce a large-scale annotated mechanical components benchmark for classification and retrieval tasks named Mechanical Components Benchmark (MCB): a large-scale dataset of 3D objects of mechanical components. The dataset enables data-driven feature learning for mechanical components. Exploring the shape descriptor for mechanical components is essential to computer vision and manufacturing applications. However, not much attention has been given on creating annotated mechanical components datasets on a large scale. This is because acquiring 3D models is challenging and annotating mechanical components requires engineering knowledge. Our main contributions are the creation of a large-scale annotated mechanical component benchmark, defining hierarchy taxonomy of mechanical components, and benchmarking the effectiveness of deep learning shape classifiers on the mechanical components. We created an annotated dataset and benchmarked seven state-of-the-art deep learning classification methods in three categories, namely: (1) point clouds, (2) volumetric representation in voxel grids, and (3) view-based representation.
"machine" - Google News
November 13, 2020 at 04:08AM
https://ift.tt/3eSejCO
Machine learning for making machines: Applying visual search to mechanical parts - Purdue News Service
"machine" - Google News
https://ift.tt/2VUJ7uS
https://ift.tt/2SvsFPt
Bagikan Berita Ini
0 Response to "Machine learning for making machines: Applying visual search to mechanical parts - Purdue News Service"
Post a Comment