
The year 2021 will see KM back in fashion, something I have discussed in previous columns, as the use of machine learning/AI allows us to finally get a grip on mountains of information and to identify and make use of valuable knowledge assets. Products are either on the market or coming to market that follow through on that promise. But before we get too carried away, it’s worth pointing out that KM will be just as dependent on human knowledge and insights as ever; machine learning/AI will augment the work of humans, not replace it. That’s important as, in reality, machine learning/AI will replace workers in many other areas, but not in KM.
Fundamentally, there is a sea change underway when it comes to the use of enterprise AI. Just a few years back, we were assailed by IBM marketing Watson, and of course, when it won JEOPARDY!, it was big news. AI was marketed as superintelligence—smarter than humans—and more powerful. As early adopters discovered, though, “artificial” also means “fake”; the system isn’t brilliant as such. Instead, it is astute at learning to do specific tasks. The efficacy of those now- automated tasks is predicated on the data that the system learned with. AI/machine learning is not so smart that it knows good data from bad; if it learns via bad data, it will misbehave. AI works well when it is fed high-quality, clean data and is supervised closely by humans. Machines learn, but they need humans to teach.
A new realization
In a sense, what we are witnessing is a denouement of sorts, a dawning realization that AI may be mighty, but that it is not as “intelligent” as some might like to think. In the world of AI, the term “humans in the loop” (HITL) is widely used. It simply means that humans check and provide feedback on the accuracy or quality of any machine learning system’s output. Some AI developers aim to take humans out of the loop, though, increasingly, many others want to bring humans more fully into the process.
The new breed of KM systems leverages machine learning but relies on humans to manage the systems very closely. Take, for example, creating a knowledge card, a summary document of a topic or theme. Read this card, and you will have a basic understanding of what you need to know and a starting point to dive deeper into the topic. Similar to Google, consumer search engines have been doing that for years now—at the top of your search for a famous actor or even a less well-known one like myself, for example, you will see a synopsis. In my case, it tells you that I am the founder of Deep Analysis and lists some movies I have been in and books I have authored. Search engines such as Google have access to massive volumes of data and can automate that task completely with machine learning. But when we are talking about organizational knowledge on a topic, that approach won’t work. In these organizational situations, you need people who understand the nuances, relevance, and organization.
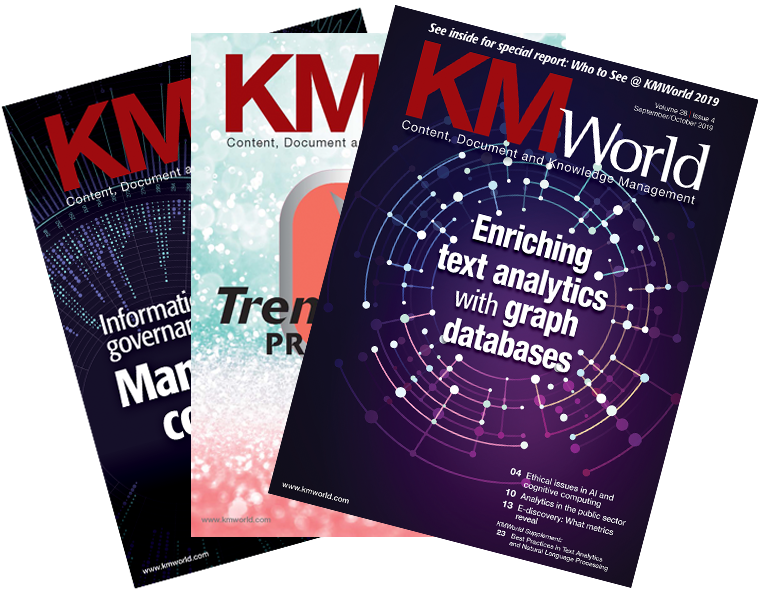
"machine" - Google News
January 11, 2021 at 12:00PM
https://ift.tt/38tDnhT
The rise of machine teaching - KMWorld Magazine
"machine" - Google News
https://ift.tt/2VUJ7uS
https://ift.tt/2SvsFPt
Bagikan Berita Ini
0 Response to "The rise of machine teaching - KMWorld Magazine"
Post a Comment