In the mid-2000s, the compressed air system at the Toyota North America plant in Georgetown, KY, crashed on average more than once a year. That led some at the plant to wonder whether leveraging machine learning and AI could address this problem by providing anomaly detection, fault identification and, most importantly, prediction of impending failures before they occur.

Unplanned downtime of the compressed air system meant, of course, that the entire factory had a problem. The paint shop used compressed air to spray paint new vehicles. The system brought air tools to life, and nothing compared to compressed air for blowing off sanding and other residues.
With a finished vehicle rolling off one of three lines at the plant every 25 seconds during two shifts, consequences were significant if the compressed air utility failed.
“The expense, if the line goes down, is tremendous,” said Mark Rucker, project manager and electrical subject matter expert at the plant. “So, there’s a good incentive there to have a good, reliable, stable utility.”
The multi-million-dollar compressed air system is comprised of a dozen Ingersoll Rand centrifugal air compressors, each the size of a small semi-truck. The end-stage blades of each compressor rotate at 20,000 rpm a fraction of an inch from their housing. If a blade operates the least bit out of spec and touches the housing, it’s a goner. The entire machine must be rebuilt at a cost of about $100,000.
The blade’s wobble isn’t the cause of a crash, though; it’s the result.
The crash—a.k.a. a surge or reverse flow—happens when something causes the normal flow of air from the compressor to a pipe try to reverse itself and the air in the pipe pushes back.
The frequency, cost and consequences of crashes prompted Toyota to call Jay Lee, founding director of Intelligent Maintenance Systems, for help.
Jay Lee was ahead of the Industry 4.0 curve
At the start of the new millennium, prior to the call from Toyota, Lee was an engineering professor at the University of Cincinnati who envisioned all the machines in a factory connected and providing data for intelligent maintenance. At that time, though, much of the technology needed to make Lee’s vision a reality didn’t yet exist.
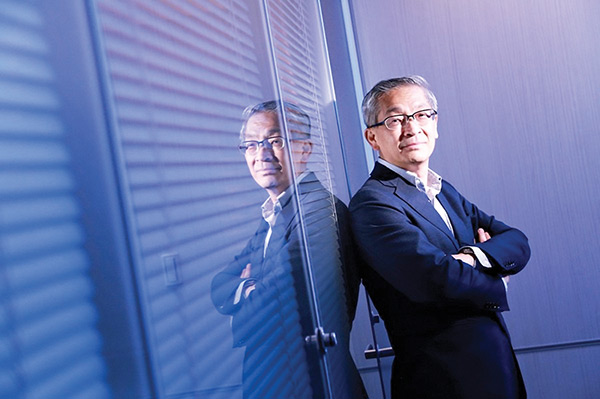
“Twenty years ago, very few people believed it was possible,” said Lee, who’s currently on leave from teaching and is vice chairman and board member of Foxconn Technology Group in Wisconsin.
In his industrial vision, machines are like patients and data collected from sensors that monitor machines provide information about their health. Experts derive prognoses by interpreting the data they collect and analyze.
“I said, ‘Why not test the machine’s blood?’ And what’s the machine’s blood?” Lee said. “Data. The question is, ‘What data do you want to get?’ It depends on what you want to analyze. We need to understand the context and content, and further formulate a relationship model among the invisible unknowns.”
Hidden in the data is meaning about deviant behavior of a machine or a process. Unlocking that meaning leads to predicting quality, determining the stability of the machine or process and preventing potential failures. “That’s exactly what Industry 4.0 is about today,” Lee said.
The term “Industry 4.0,” of course, wasn’t popularized until around the time of the German government’s initiative in 2013.
No crashes since 2006
Once at Toyota, Lee and his team of data analysts joined with Rucker and a group of skilled maintenance workers and plant engineers to tackle the compressed air system problem.
The simplicity of their questions belied the complexity of the task ahead.
“Can we predict a reverse-flow problem before it causes a crash?” Rucker said. “Can we see something developing in time to do something about it?”
Through months of trial-and error in 2005, the team collected and analyzed high-fidelity data from one of the compressors and looked for anomalies. Sometimes the team would create a surge or approach-to-surge condition so they could force the machine to start to exhibit deviant behavior and see what that looked like in the data set.
Eventually, the team’s work paid off. Its members saw the best predictor of an incipient surge condition occurred at stage two (of the four stages the compressor runs through that progress from normal pressure to 120 pounds of pressure per square-inch, or PSI).
“What are the physics behind that, why is this the predictor?” said Rucker. “Don’t know, don’t care. We just saw that this reliably predicts a future surge, and it predicts it with seconds of warning.”
The next step was to put a differential sensor on each compressor and create a machine-learning algorithm for the central control unit. Tests confirmed that as soon as stage two started to “wobble,” the compressor would start backing off.
“Every time we tested it, it kept us out of trouble,” he said. “We’ve had no crashes since 2006.”
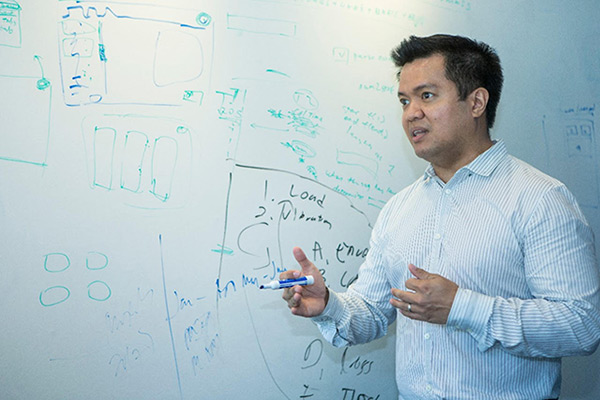
While the compressor-surge project was a success, another that focused on trying to predict the life of bearings in the compressed air behemoths failed. The team couldn’t find enough reliable maintenance data and pair it with machine operational data to draw any useful correlations, Rucker said.
“That’s probably about the right mix of R&D,” he said. “You try stuff, and you don’t know if it’s going to work or not. And if half the time you get your money back, that’s a win. And every so often you find that one that pays for everything else—like the surge control.”
Flexibility is key
Lee subsequently published a paper about the Toyota project and, in 2013, helped co-found Predictronics with Patrick Brown, an employee at Intelligent Maintenance Systems, and two of his university students, David Siegel and Edzel Lapira. Brown. Lapira and Siegel form the Predictronics C suite.
Like Toyota’s Rucker called on Lee for AI help, companies come to Predictronics for help with their zero-downtime aspirations.
“Different customers are usually at different levels of maturity,” said Lapira, who is CEO. “We have some customers who already have a data-collection structure in place: They have a data lake but they don’t know what to do with the data. We also have some customers who have to start from scratch.”
Customers with 50- or 60-year-old assets must start at an even earlier stage.
Increasingly, Predictronics is approached by customers with legacy machines. Lapira and his colleagues send them shopping for components like accelerometers and transducers.

“These are the different analog-to-digital converters we need to get that data out,” he said.
Since its founding in 2013, Predictronics has helped more than 70 factories with AI solutions, many of them Fortune 500 companies.
Predictronics worked with one customer, a semiconductor manufacturer, to establish a virtual metrology predictive solution that was able to learn the relationship between sensor and measurement variables.
The project was challenging due to the hundreds of measured signals thrown off by the semiconductor equipment.
But there are well-known statistical methods for narrowing down the number of possibilities, Siegel said.
“In addition to those methods, over the last several years it’s been more of the machine learning community that’s developed methods that incorporate a classification regression algorithm that have a built-in variable selection part of it,” he said. “So that narrows it down to the top 10, or 20 or 30 variables. Then you work with the customer or use your own knowledge to determine what makes the most sense to include in the software and the analysis model.”
Whether Predictronics applies knowledge gained from previous experience in its template-based approach, which helps keep costs down, or a solution formulated in one part of a company that can then be scaled in-house, “finding a solution that directly addresses the most critical issues but is also flexible enough to be applied to other problems is key,” Brown said.
Due diligence AI
Factories that are shopping around for an AI solutions provider like Toyota did for its compressed air problem should do their due diligence to find just the right fit.
It helps to know the right questions to ask, said the experts at Predictronics:
Where have your services been used before?
Has your solution demonstrated a clear improvement on the business operations in terms of reduced downtime or increased quality?
Has your solution been used in my industry?
Does your team have industrial domain knowledge?
Does your solution work with legacy equipment?
Does your solution work with a variety of sensors?
Does your solution use machine learning?
How much data does it require for training the model?
Does it need just data from a healthy machine or data from a degraded or faulty machine also to learn from?
Robots want AI, Too
While the machine tools in a shop are performing better under AI’s influence, there’s no reason why robots can’t do the same.
Fanuc America recently added “AI Error Proofing” to its robot controller and the robots’ vision function, iRVision, which uses machine learning (ML) for part inspection.
With the error-proofing capability, an operator uses images to train the ML to check for two distinct situations—if a welded nut is present or absent, for example—and accept or reject a part.
Because iRVision can support as many as 27 cameras, the error-proofing function can act at as many points in the manufacturing process.
“You want to identify rejects or errors before you continue to add value to a bad part,” said Josh Person, staff engineer in Fanuc’s machine vision group.
“Often, you can see an error at one stage and the next stage would cover it up,” he added.
During setup, the operator can present multiple examples of work pieces and classify them into two categories—good and bad. If the example doesn’t fall into either class, it will output “undetermined.” Undetermined examples can then be added to improve the learned model.
"machine" - Google News
September 29, 2021 at 08:03PM
https://ift.tt/2WrIRqm
Go for zero-downtime performance by 'testing the machine's blood' - Advanced Manufacturing
"machine" - Google News
https://ift.tt/2VUJ7uS
https://ift.tt/2SvsFPt
Bagikan Berita Ini
0 Response to "Go for zero-downtime performance by 'testing the machine's blood' - Advanced Manufacturing"
Post a Comment