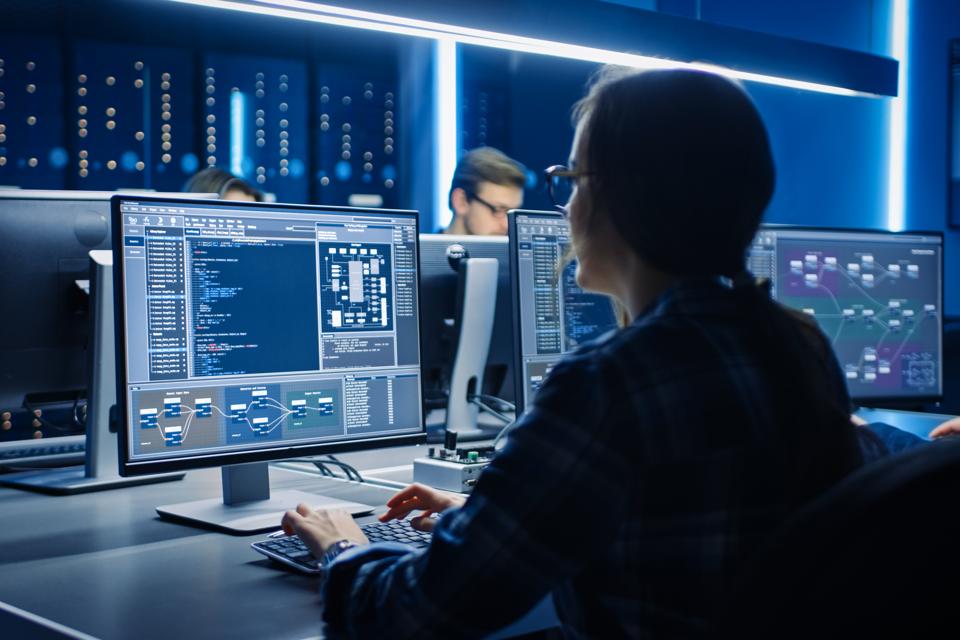
getty
- Before Covid-19 financial institutions saw a 10:1 ratio of bot-based malicious to legitimate login attempts, according to Aite Group's Fraud & AML practice. Malicious login attempts are setting new records every month.
- Between 2018 and 2019, there was an 84% increase in the number of breached data reports, reaching 15.1B accounts last year.
- Fraud operations funded by organized crime run much like legitimate businesses, complete with ongoing recruiting campaigns for AI, bot and machine learning expertise and office locations focused on developing breach strategies.
- As of June 2020, login credentials for online banking averaged about $35 on the dark web while payment card details averaged between $12 and $20 apiece, according to analysis again by Help Net Security.
Interested in understanding how AI and machine learning are being used to prevent bot-based fraud attempts, I attended a few recent webinars with Kount's 3 Key Elements Needed For Successful Bot Detection being one of the most insightful. Trace Fooshee, Senior Analyst at Aite Group and Sven Hindman, Product Manager at Kount, have decades of expertise in this area. The webinar provided an opportunity to see what's new in using AI and machine learning to prevent fraud. The following key insights from the webinar reflect how advanced bot-based fraud is and the three steps needed to detect better and thwart fraud-based bot attacks:
- Fraud rings have grown in complexity and scale and resemble enterprises today, complete with their financial crime value chain. Trace Fooshee, Senior Analyst at Aite Group, created a financial crime value chain framework that explains the enterprise-level scale bot-based fraud strategies in use today. Fraud rings rely on bad bots to accomplish the goals of every phase of the value chain, starting with mining raw materials or card, credential, password and personal data. Each phase of the value chain is powered by the data bad bots capture every day.
- The bot landscape is changing fast as fraudsters look to capitalize on the confusion, fear and uncertainty surrounding Covid-19 and its immediately accelerating e-commerce. Covid-19 quickly became the catalyst that e-commerce and digital transformation initiatives needed to prove they could scale. It also led to a massive increase in bot attacks. The following graphic explains the ratio of malicious login attempts to legitimate ones using a curve that progresses to the left. Trace Fooshee, Senior Analyst at Aite Group, says the figure below was the baseline before Covid-19; now, it's exponentially greater in terms of malicious login attempts.
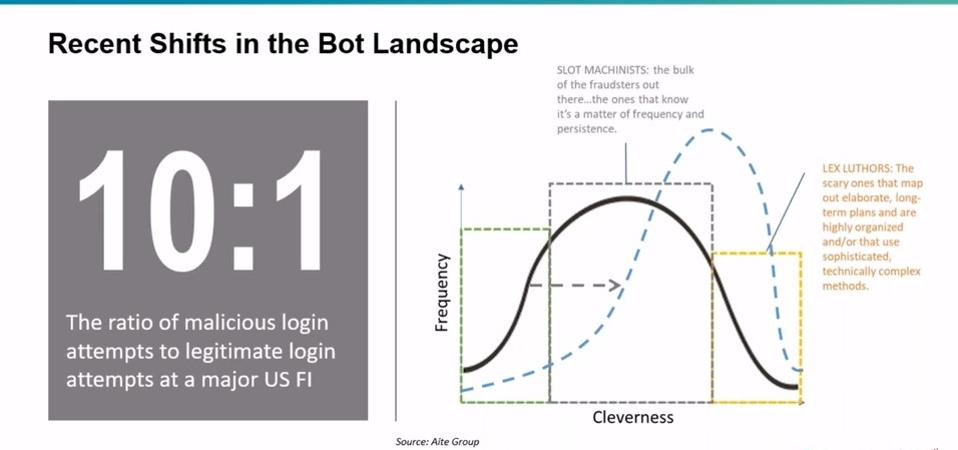
Kount's 3 Key Elements Needed For Successful Bot Detection webinar
- The three steps to better bot detection using AI and machine learning include analyzing all available data in the Identity Trust Global Network, using AI and machine learning to detect suspicious bad bot activity and responding to the threat in real-time. Kount's approach to using AI and machine learning is predicated on having supervised and unsupervised machine learning algorithms iteratively "learn" fraud patterns over time. Kount relies on its Identity Trust Global Network to calculate Identity Trust Levels in milliseconds, reducing friction, blocking fraud and delivering improved user experiences. The Identity Trust Global Network includes more than a decade of trust and fraud signals built across industries, geographies and 32B annual interactions, combined with expertise in AI and machine learning to turn trust into a sales and customer experience multiplier. The following is the first of three steps in using machine learning to achieve better bot detection:
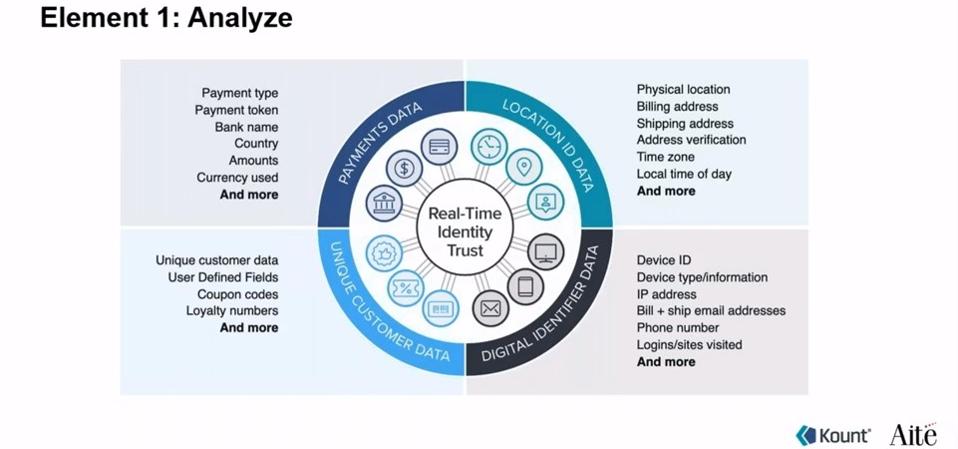
Kount's 3 Key Elements Needed For Successful Bot Detection
- Combining the innate strengths of unsupervised and supervised machine learning to provide a Transaction Safety Rating is the second step in using machine learning to thwart bot-based fraud. Reducing the uncertainty of a given transaction in milliseconds take machine-learning-based insights from supervised and unsupervised algorithms that provide instinctual and experiential-based data. The combination of each based on the Identity Trust Global Network helps contribute to a more frictionless buying experience, fewer false-positives and greater customer loyalty over time because business becomes easier to buy from. The following graphic illustrates how Transaction Safety Ratings are created:

Kount's 3 Key Elements Needed For Successful Bot Detection webinar
- The third step uses the outcome of AI and machine learning to define the most appropriate response based on trust levels customized for a specific business and its requirements. Of the many AI and machine learning-based companies focused on fighting fraud, Kount's approach of defining an adaptive trust level configurable by individual policies is unique. Knowing adaptive trust levels is especially helpful for tailoring selling strategies seasonally while still focusing on thwarting advanced bot fraud attempts.
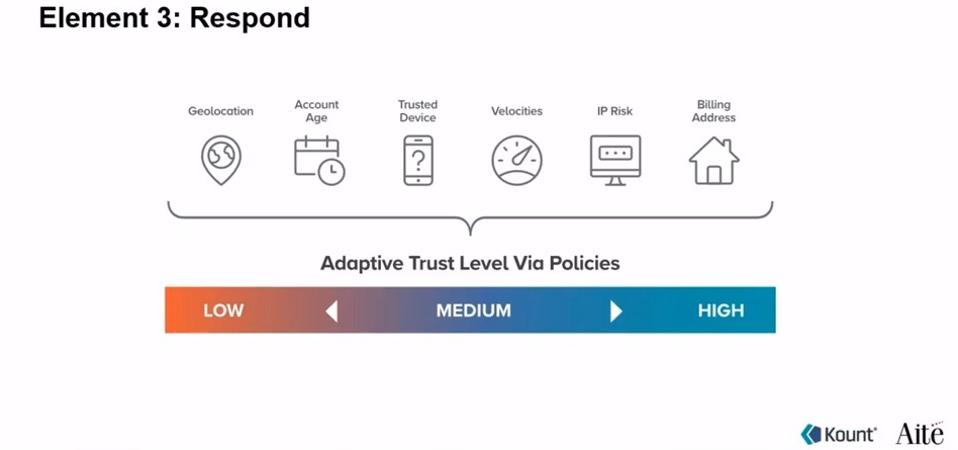
Kount's 3 Key Elements Needed For Successful Bot Detection
"machine" - Google News
September 28, 2020 at 01:16AM
https://ift.tt/2S35347
How To Improve Bot Detection With Machine Learning - Forbes
"machine" - Google News
https://ift.tt/2VUJ7uS
https://ift.tt/2SvsFPt
Bagikan Berita Ini
0 Response to "How To Improve Bot Detection With Machine Learning - Forbes"
Post a Comment