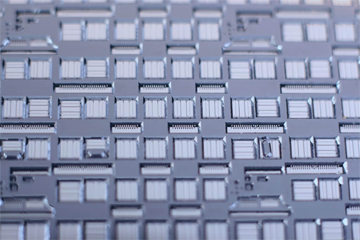
Recently, STMicroelectronics released a digital inclinometer with a machine learning core. What features does the IIS2ICLX include, and how does the machine learning core help designers?
What is an inclinometer?
An inclinometer is a device that can measure the angle of a slope with respect to gravity. Historically, inclinometers have come in a range of shapes and sizes that utilises different mechanisms to measure inclination. One such method involves a compass suspended in a fluid that, when tilted, will push the needle up or down. Another method involves the use of a protractor and sight with a small weight, the weight on a string shows the direction of gravity, and the protractor is sighed along with the tilt in question, and the resulting reading on the protractor determines the tilt.
The world of MEMS has allowed for a range of different sensors to be constructed for use in electronic circuits, and inclinometers are no exception. Modern digital inclinometers make use of 2-axis accelerometers that allow for the tilt to be measured in two plains, as well as being able to detect vibration and apply calibration.
What is machine learning?
Machine learning is the ability for a system to recognise patters from a source to produce an output, while also being able to learn and adapt to the output to better improve its performance. Machine learning, often referred to as Artificial Intelligence (AI), is quickly becoming an important ability in modern electronics as it allows for complex tasks to be easily achieved without the need for programming all possibilities. A classic example of how machine learning can be beneficial is speech recognition. Speech recognition could, in theory, be coded with a large number of comparative statements that compares each word in a sentence to every single word in the dictionary, said by every different type of person (accents, gender, age etc.). However, this would require resources beyond any computing system, and would take an aeon to process. Instead, machine learning allows for a neural net to be trained to recognise patterns, and thus does not require complete information to make an accurate determination. Each time a machine learning algorithm hears a new way of saying a word, it makes slight internal adjustments to ensure that upon hearing the same word again, the correct response is produced. Thus, a machine learning system would look for patterns in phonetics and timing as opposed to comparing two specific audio files.
STMicroelectronics launches the IIS2ICLX
Recently, STMicroelectronics launched their latest digital inclinometer, the IIS2ICLX, a two-axis accelerometer with a machine learning core. The IIS2ICLX utilises MEMS technology and allows for measurement over different scales of acceleration including ±0.5/±1/±2/ and ±3g. The inclinometer sensor outputs data over I2C and SPI interfaces, while internal compensation enables for a temperature stabilised sensor. High accuracy of the smart sensor is achieved thanks to the low noise density of 15μg/√Hz, and thus allows for tilt sensing as well as sensing of low-frequency vibration. The IIS2ICLX is aimed at industrial applications including antenna positioning, monitoring, platform levelling, forklifts, equipment installation, robotic systems, but the ability to record low-frequency vibration also makes it ideal for structural-health monitoring. The highly programmable device includes the ability to program both a high and low pass digital filter, utilise an internal finite state machine, deploy machine learning routines, and store up to 3KB of internal data in a FIFO buffer. Housed in an LGA 5 x 5 x 1.7mm package, the sensor has an operating temperature range of -40°C to +105°C, is RoHS and Green compliant, and has high shock survivability making it highly ideal for any industrial application.
What does the machine learning core do?
The internal machine learning core in the IIS2ICLX is a pattern recognition system that can greatly help benefit applications such as Industry 4.0. The machine learning core allows for a set of predetermined user classes to be programmed in and watched for in data readings from the inclinometer sensor. For example, the nominal operation of a piece of equipment would produce a specific pattern of vibration, and this would be ignored by the sensor. If, however, the equipment under monitor began to experience a failing motor, the vibration sensor would produce anomalous readings, thus triggering the machine learning core of the sensor. The machine learning core in the IIS2ICLX can run up to 8 flows instantaneously, with each flow having up to 256 possible results.
How will machine learning change the future in sensors?
The reduction in transistor size, coupled with the falling prices of electronics is seeing even sensors becoming increasingly powerful. Eventually, sensors themselves will be fitted with microcontrollers and other supporting hardware that can help to offload complex tasks from the main controller, but also help to reduce overall power consumption. Sensors that can pre-process data and make decisions will greatly reduce the complexity of a system, and be highly beneficial to Industry 4.0, IoT, and IIoT. The use of machine learning in sensors also helps to reduce the latency of systems whereby sensors can immediately react to data as it is recoded, instead of being streamed to a central controller to then be processed. The future of sensors may see direct bridging between sensors and actuators which could do entirely without the use of the main processing unit (in a similar fashion to involuntary reactions in the human nervous system).
Read More
"machine" - Google News
September 02, 2020 at 03:54PM
https://ift.tt/3hRB8ax
STMicroelectronics Launches IIS2ICLX Digital Inclinometer with Machine Learning - Electropages
"machine" - Google News
https://ift.tt/2VUJ7uS
https://ift.tt/2SvsFPt
Bagikan Berita Ini
0 Response to "STMicroelectronics Launches IIS2ICLX Digital Inclinometer with Machine Learning - Electropages"
Post a Comment