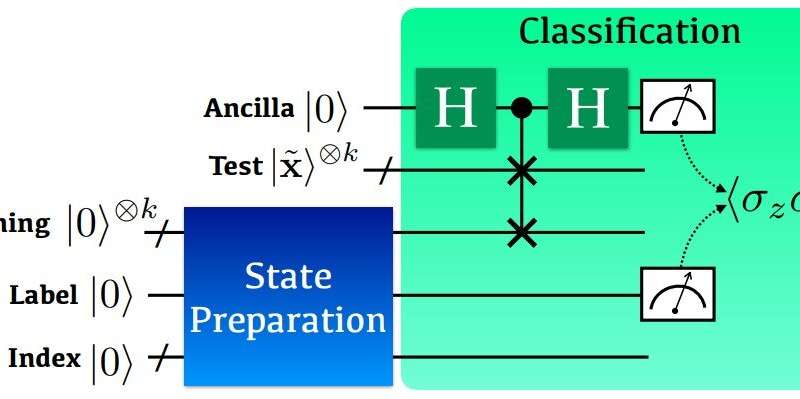
Quantum information scientists have introduced a new method for machine-learning classifications in quantum computing. The non-linear quantum kernels in a quantum binary classifier provide new insights for improving the accuracy of quantum machine learning, deemed able to outperform the current AI technology.
The research team led by Professor June-Koo Kevin Rhee from the School of Electrical Engineering, proposed a quantum classifier based on quantum state fidelity by using a different initial state and replacing the Hadamard classification with a swap test. Unlike the conventional approach, this method is expected to significantly enhance the classification tasks when the training dataset is small, by exploiting the quantum advantage in finding non-linear features in a large feature space.
Quantum machine learning holds promise as one of the imperative applications for quantum computing. In machine learning, one fundamental problem for a wide range of applications is classification, a task needed for recognizing patterns in labeled training data in order to assign a label to new, previously unseen data; and the kernel method has been an invaluable classification tool for identifying non-linear relationships in complex data.
More recently, the kernel method has been introduced in quantum machine learning with great success. The ability of quantum computers to efficiently access and manipulate data in the quantum feature space can open opportunities for quantum techniques to enhance various existing machine learning methods.
The idea of the classification algorithm with a nonlinear kernel is that given a quantum test state, the protocol calculates the weighted power sum of the fidelities of quantum data in quantum parallel via a swap-test circuit followed by two single-qubit measurements (see Figure 1). This requires only a small number of quantum data operations regardless of the size of data. The novelty of this approach lies in the fact that labeled training data can be densely packed into a quantum state and then compared to the test data.
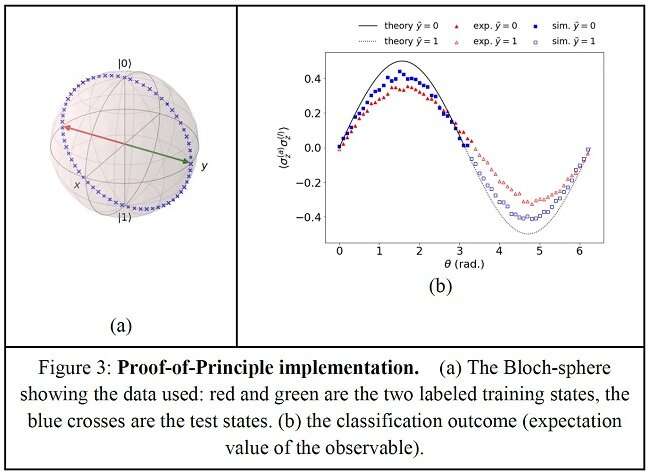
The KAIST team, in collaboration with researchers from the University of KwaZulu-Natal (UKZN) in South Africa and Data Cybernetics in Germany, has further advanced the rapidly evolving field of quantum machine learning by introducing quantum classifiers with tailored quantum kernels.
The input data is either represented by classical data via a quantum feature map or intrinsic quantum data, and the classification is based on the kernel function that measures the closeness of the test data to training data.
Dr. Daniel Park at KAIST, one of the lead authors of this research, said that the quantum kernel can be tailored systematically to an arbitrary power sum, which makes it an excellent candidate for real-world applications.
Professor Rhee said that quantum forking, a technique that was invented by the team previously, makes it possible to start the protocol from scratch, even when all the labeled training data and the test data are independently encoded in separate qubits.
Professor Francesco Petruccione from UKZN explained, "The state fidelity of two quantum states includes the imaginary parts of the probability amplitudes, which enables use of the full quantum feature space."
To demonstrate the usefulness of the classification protocol, Carsten Blank from Data Cybernetics implemented the classifier and compared classical simulations using the five-qubit IBM quantum computer that is freely available to public users via cloud service. "This is a promising sign that the field is progressing," Blank noted.
Explore further
Citation: Scientists introduce new method for machine learning classifications in quantum computing (2020, July 7) retrieved 7 July 2020 from https://ift.tt/3iH13Td
This document is subject to copyright. Apart from any fair dealing for the purpose of private study or research, no part may be reproduced without the written permission. The content is provided for information purposes only.
"machine" - Google News
July 08, 2020 at 01:48AM
https://ift.tt/3iH13Td
Scientists introduce new method for machine learning classifications in quantum computing - Phys.org
"machine" - Google News
https://ift.tt/2VUJ7uS
https://ift.tt/2SvsFPt
Bagikan Berita Ini
0 Response to "Scientists introduce new method for machine learning classifications in quantum computing - Phys.org"
Post a Comment