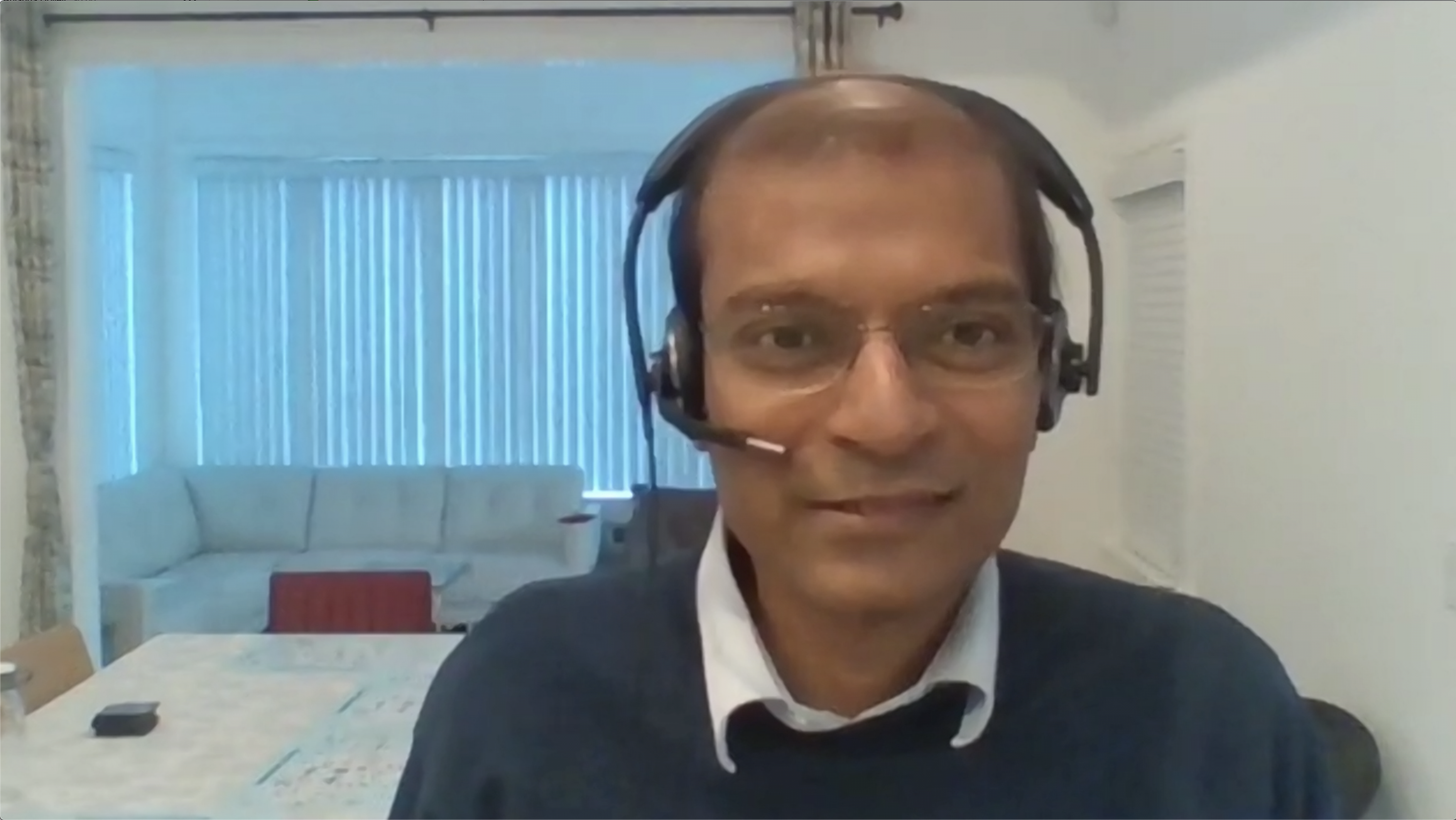
Machine learning has experienced an incredible increase in usage in the past couple of years. In 2017, deploying ML models was considered extremely difficult, and something only major organizations had the resources to consider.
Then, Amazon Web Services Inc. released the Amazon SageMaker machine learning service.
“Customers saw it was much easier to do machine learning once they were using tools like SageMaker,” said Bratin Saha (pictured), vice president and general manager of machine learning services and Amazon AI at Amazon. “Machine learning was no longer niche; machine learning was no longer a fictional thing. It was something that was giving real business value.”
Saha spoke with Dave Vellante, host of theCUBE, SiliconANGLE Media’s livestreaming studio, during AWS re:Invent. They discussed trends in machine intelligence learning and artificial intelligence and how they are supported by Amazon SageMaker. (* Disclosure below.)
SageMaker makes machine learning accessible to every business
As customers saw the value in machine learning, they went from deploying tens of models to deploying hundreds of thousands of models, making SageMaker one of the fastest-growing services in AWS history, according to Saha.
“Today, we have one customer who is deploying more than a million models,” he said.
But as usage grew, so did the problems customers were reporting. So, AWS grew SageMaker in response. More than 50 new capabilities have been added to SageMaker in just the past year, and re:Invent 2020 saw the addition of nine more.
Running through these in his discussion with theCUBE, Saha described each in detail and gave key use cases. For example, SageMaker Data Wrangler is an addition to SageMaker Studio, a fully integrated environment for machine learning. By giving customers the ability to do data preparation in the same service as machine learning, it reduces the time spent cleaning data.
“With a few clicks you can connect to a variety of data stores … and do all of your data preparation,” Saha stated. “You get your data in; you do some interactive processing. Once you’re happy with the results of your data, you can just send it off as an automated data pipeline job. It’s the easiest and fastest way to do ML and take out that 80% [of time wrangling data].”
Another new service is Amazon SageMaker Clarify, which provides visibility and transparency into the modeling process in order to eliminate unintentional biases.
“[Clarify] helps to convert insights that you get from model predictions into actionable insights because you now know why the model is predicting what it is predicting,“ Saha said.
Distributed Training on Amazon SageMaker increases the efficiency for customers training really large models, some of which now have billions of parameters, according to Saha. The new capability uses two techniques, model parallelism and data parallelism. The first enables these massive models that could take weeks to train on a single graphics processing unit to be trained in parallel across multiple GPUs. The second takes models that are too large to fit in the memory of a single GPU and automatically distributes them across multiple GPUs.
These capabilities are “making it much faster and much easier for customers to work with large models,” Saha said.
Bringing machine learning to edge devices and helping fulfill Amazon’s mission of “AWS Everywhere” is Amazon SageMaker Edge Manager. Optimizing models so they can run on an edge device increases the benefit by 25x, according to Saha. Not only does Edge Manager enable local inference, but it helps maintain model quality.
“Once deployed it monitors quality of the models by letting you upload data samples to SageMaker so you can see if there is drift in your models or any other degradation,” Saha stated.
For complete descriptions and discussion on all the new Amazon SageMaker capabilities, watch the complete video interview below. Then be sure to check out more of SiliconANGLE’s and theCUBE’s coverage of AWS re:Invent. (* Disclosure: Amazon Web Services Inc. sponsored this segment of theCUBE. Neither AWS nor other sponsors have editorial control over content on theCUBE or SiliconANGLE.)
Photo: SiliconANGLE
Since you’re here …
Show your support for our mission with our one-click subscription to our YouTube channel (below). The more subscribers we have, the more YouTube will suggest relevant enterprise and emerging technology content to you. Thanks!
Support our mission: >>>>>> SUBSCRIBE NOW >>>>>> to our YouTube channel.
… We’d also like to tell you about our mission and how you can help us fulfill it. SiliconANGLE Media Inc.’s business model is based on the intrinsic value of the content, not advertising. Unlike many online publications, we don’t have a paywall or run banner advertising, because we want to keep our journalism open, without influence or the need to chase traffic.The journalism, reporting and commentary on SiliconANGLE — along with live, unscripted video from our Silicon Valley studio and globe-trotting video teams at theCUBE — take a lot of hard work, time and money. Keeping the quality high requires the support of sponsors who are aligned with our vision of ad-free journalism content.
If you like the reporting, video interviews and other ad-free content here, please take a moment to check out a sample of the video content supported by our sponsors, tweet your support, and keep coming back to SiliconANGLE.
"machine" - Google News
December 11, 2020 at 07:27AM
https://ift.tt/3qFe17M
Amazon SageMaker grows ever more powerful as machine learning models head for the edge - SiliconANGLE News
"machine" - Google News
https://ift.tt/2VUJ7uS
https://ift.tt/2SvsFPt
Bagikan Berita Ini
0 Response to "Amazon SageMaker grows ever more powerful as machine learning models head for the edge - SiliconANGLE News"
Post a Comment