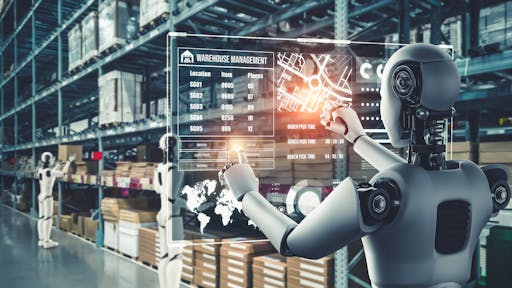
Blue Planet Studio/stock.adobe.com
In a mid-2020 issue of Supply & Demand Chain Executive, I had the pleasure of speaking with managing editor Brielle Jaekel on the “emerging technologies that claim to help companies in the supply chain.” During that conversation, I mentioned the evolving role of artificial intelligence (AI) and machine learning (ML):
“In the near future, supply chain AI will begin to migrate to machine learning. Currently, supply chain AI consists of developers programming business rules, telling computers what to look for and what action to take when it encounters those situations, but as AI migrates to machine learning, it will begin to think for itself. As machine learning becomes more advanced, technologies will increasingly be able to make note of repetitive situations and past experiences to start learning and making recommendations on its own. Technology like this has already deployed on a wide scale in other industries, and it has the potential to rapidly automate and improve a wide range of supply chain processes.”
A year and half later, it’s time to re-examine how companies adopted these technologies to optimize their supply chain processes and determine how they could continue to evolve.
Tech adoption on the rise
The supply chain challenges of the last couple years, brought on by the global pandemic, extreme weather events, Brexit, and labor shortages, caused many companies to examine their strategies and invest in technologies to help bypass disruptive bottlenecks. According to The Wall Street Journal:
“Shipping bottlenecks and shortages of everything from semiconductors to chicken wings are drawing more attention to technology aimed at streamlining supply chains and boosting efficiency in distribution networks. Companies are also looking to automation and software to help tamp down rising logistics costs and meet growing demand for e-commerce and delivery services.”
AI, and therefor ML, adoption lags behind
As Harvard Business Review reported in September 2021, AI adoption by business has skyrocketed since the start of the pandemic, but adoption for supply chain issues is still in its infancy:
“These are still the early days for AI-driven supply chains, a survey released by the American Center for Productivity and Quality finds only 13% of executives foresee a major impact from AI or cognitive computing over the coming year. Another 17% predict a moderate impact. Businesses are still relying on manual methods to monitor their supply chains — those that adopt AI in the coming months and years will achieve significant competitive differentiation.”
Why the reticence?
While the challenges of the last couple years exposed many problems with companies’ supply chain processes, the efforts to address them have been plagued by current-thinking rather than forward-thinking. Investment in technologies that address specific immediate problems often supersede investment in technologies that could prevent problems down the road. In some ways that makes sense: plug the hole letting water into the boat before worrying about propulsion to shore. The problem comes when companies focus only on solving the current problem. Once the hole in the boat is plugged to keep it from sinking, paddling the boat against the tide makes for slow progress and leaves you vulnerable to being passed by the competition who have outboard motors.
Machine learning offers a leg up
As noted by Harvard Business Review, “those that adopt AI in the coming months and years will achieve significant competitive differentiation.” As AI becomes ML, it can optimize supply chain operations in ways that manual processes simply cannot. By taking in large volumes of data, ML can rapidly spot trends and make recommendations for immediate improvement, whether the global supply chain is still bogged down by bottlenecks or not. It can learn, optimize, inform, simplify, verify, and scale, all in a much more time and cost-efficient manner.
Every organization creates enormous amounts of data with every supply purchase, manufacturing order, warehouse and retail location restock, customer interaction, sale, order shipment and return. Taking even a small portion of that – order shipments, for example – creates large data volumes, including:
- Execution-based data. Quantitative data, such as shipment cost, carrier service levels, carton dimensions, carton weight, carton counts, carton origins, and carton destinations.
- Track and trace data. Delivery status messages for each tracking number from the point of shipment manifest through delivery and, in the case of returns, back again.
- Control Tower data. Carrier and service level performance, issue frequency, employee performance, and associated factors.
For organizations shipping thousands, tens of thousands or even hundreds of thousands of parcels per day, the data volume can easily overwhelm and bog down manual analysis. Add in the data from each other point in the supply chain, and it becomes apparent how easily opportunities for optimization can get overlooked. Machine learning can reduce that likelihood and ease the burden on staff.
If not now, when?
If the analysis reported by Harvard Business Review plays out as predicted, less than 30% of executives foresee AI and ML having any kind of impact in the immediate future. That’s a giant, flashing red light signaling a significant advantage for forward-thinking organizations. The investment in supply chain technology is already booming, but it’s focused on short-term needs. When the organizations only investing in plugging the holes in their boat look up, they’re likely to find the competition has left them in their wake.
"machine" - Google News
February 21, 2022 at 09:08PM
https://ift.tt/8STQxYN
Machine Learning in the 2022 Supply Chain - Supply and Demand Chain Executive
"machine" - Google News
https://ift.tt/wQ3lBtF
https://ift.tt/Y01LtFJ
Bagikan Berita Ini
0 Response to "Machine Learning in the 2022 Supply Chain - Supply and Demand Chain Executive"
Post a Comment