The intended purpose of machine learning (ML) in cardiovascular medicine is to help guide clinical diagnoses as well as promote scientific discovery. Whether ML is implemented by most clinical cardiologists and cardiovascular researchers will likely depend on the successful resolution of concerns fueling hesitancy to embrace ML. This commentary discusses caveats related to ML in clinical practice, and offers suggestions for stakeholders on how to bridge knowledge gaps and clinicians’ misgivings to bring this powerful approach to the clinic to improve care of the patients we serve.
This is a preview of subscription content
Access options
Subscribe to Journal
Get full journal access for 1 year
We are sorry, but there is no personal subscription option available for your country.
Buy article
Get time limited or full article access on ReadCube.
$32.00
All prices are NET prices.
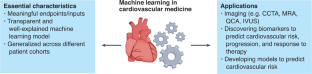
References
-
Benjamens, S., Dhunnoo, P. & Meskó, B. npj Digit. Med. 3, 118 (2020).
-
Zekavat, S. M. et al. Circulation 145, 134–150 (2022).
-
Zech, J. R. et al. PLoS Med. 15, e1002683 (2018).
-
Reardon, S. Nature 576, S54–S58 (2019).
-
Huisman, M. et al. Eur. Radiol. 31, 7058–7066 (2021).
-
Nikpay, M. et al. Nat. Genet. 47, 1121–1130 (2015).
-
Pattarabanjird, T. et al. Genes 11, 1446 (2020).
-
Ridker, P. M. et al. N. Engl. J. Med. 377, 1119–1131 (2017).
Author information
Affiliations
Corresponding author
Ethics declarations
Competing interests
The authors declare no competing interests.
Rights and permissions
About this article
Cite this article
Pattarabanjird, T., McNamara, C. The clinicians’ perspectives on machine learning. Nat Cardiovasc Res (2022). https://ift.tt/nqbiu7G
-
Published:
-
DOI: https://ift.tt/nqbiu7G
"machine" - Google News
February 28, 2022 at 11:04PM
https://ift.tt/8OIVYjW
The clinicians' perspectives on machine learning - Nature.com
"machine" - Google News
https://ift.tt/2EC6Ncj
https://ift.tt/B7LR2Az
Bagikan Berita Ini
0 Response to "The clinicians' perspectives on machine learning - Nature.com"
Post a Comment